Let's Party Like it's 1965
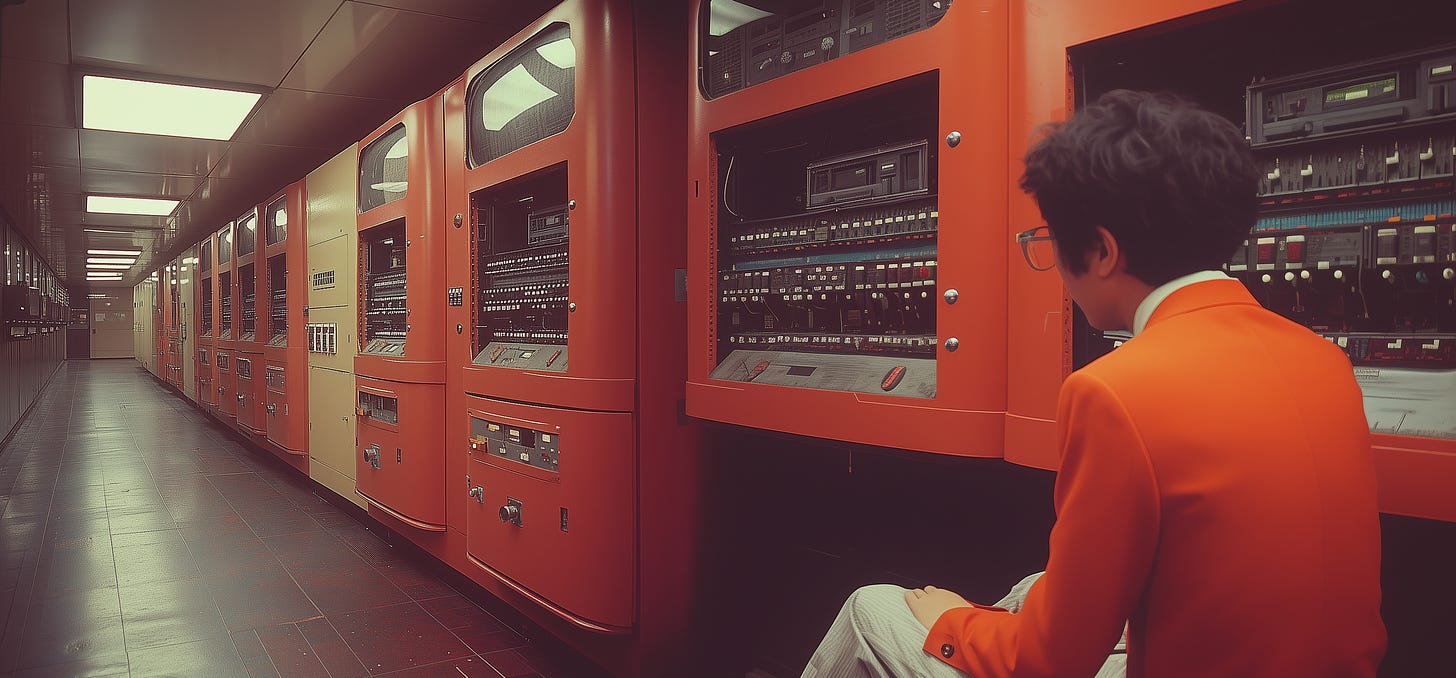
In 1965, IBM made a bold move that has since become legend in the world of technology. They committed $5 billion (about $40 billion in today’s dollars) to their System 360 mainframe line—a massive bet at a time when computers were still enormous, expensive machines used primarily by large businesses, government, and academia. And here’s the twist: there weren’t any “software developers” as we think of them today. Software engineering as a field didn’t exist yet. People with skills in mathematics, physics, and statistics found themselves writing code because they had the right analytical skills, domain expertise, and often, just the right curiosity.
Fast forward to today, and we’re seeing a similar, ambitious gamble with AI. Investors are pouring billions into AI with the hope that it will reshape society, just as computing did decades ago. However, while it’s tempting to say this is a repeat of 1965, there are crucial differences that make today’s landscape both more exciting and, in some ways, more challenging. AI may indeed be the “next computer,” but it’s a vastly more complex paradigm, one that will require a more diverse array of expertise to develop responsibly. By 2025, we’ll likely begin to see what AI will mean for the coming decades, and as with computing in the 1960s, it’s safe to say the developers of the future will be a very different breed. But the question remains: are we ready for what’s next?
IBM Goes All-In
IBM’s 1965 investment in System 360 didn’t just bring new technology to market; it laid the groundwork for modern computing. System 360 introduced concepts like modularity and compatibility across a family of machines, creating a versatile and scalable framework. This investment wasn’t just about meeting existing demand; it was about creating a future where computers were essential to every industry, from government to manufacturing. System 360 wasn’t just hardware; it was a vision for computing as an essential infrastructure for the world’s progress.
What’s striking about that era is that “software engineering” as a profession hadn’t yet taken shape. There were no degrees in computer science or formal training programs for coding. IBM recruited talent from across disciplines, pulling in mathematicians, statisticians, and scientists, often from fields unrelated to computers. These individuals weren’t hired just for technical know-how; they were chosen because they had the right mindset—analytical, inquisitive, and adaptive. The result was a new kind of workforce that was defined by problem-solving and domain expertise, and the new field of software development began to take shape around them.
System 360 proved to be a wise investment, laying down industry standards for interoperability and scalability that would shape computing for the next half-century. But it was the blend of ambitious technology and diverse human ingenuity that made it transformative. In hindsight, we can see how System 360 didn’t just introduce a new product line; it laid the foundation for computing to become ubiquitous, a backbone of modern life.
The hyperpop version of 1965?
Today, we’re seeing the same kind of enthusiasm and financial commitment surrounding AI. Companies and investors are pouring billions into everything from foundational models to autonomous systems, hoping to spark a new era. Many are calling these investments risky, or even reckless, considering that AI’s long-term potential is still largely speculative. But just as IBM couldn’t fully predict the impact of System 360, we may not yet grasp the full implications of today’s AI investments. By 2025, I believe we’ll see the first significant signs of what the coming AI era will look like.
There are critical differences between 1965 and today that need to be addressed. For one, computing had a relatively clear use case in data processing and automation, with demand coming from industries that could immediately benefit from improved efficiency. AI, on the other hand, is still far more experimental. Its applications range from narrow functions like predictive text to the complex realm of autonomous systems. Unlike IBM’s gamble on computing infrastructure, AI’s future is dependent on a range of factors that make its trajectory less predictable. For example, while hardware improvements drove computing’s exponential growth, AI faces challenges around data quality, model interpretability, and energy demands that are not as easily solved.
Nonetheless, like computing, AI’s promise is huge. If it does succeed in transforming industries, AI has the potential to become a foundational layer of modern society, reshaping fields as diverse as healthcare, education, finance, and beyond. But unlike the clear path that computing ultimately followed, AI’s journey is likely to be full of twists, turns, and ethical challenges that make this a much more complex and nuanced gamble.
It's also worth noting that–much in the same way as computers of the 1960's provided much needed computing power to corporations–we should be considering that AI is providing on-demand access to a new resource. Computing in the traditional sense is restricted to operating within narrowly defined bounds. It’s about following explicit instructions, performing calculations, and processing data according to a strict, predefined logic. A program written for a traditional computer can’t go outside the bounds set by its code; it does what it’s told, no more and no less. This isn't the case for AI however, while I am hesitant to make any bold claims about the timeline for AGI, the fact remains that we are already seeing the first signs of what generalized intelligence on-demand can deliver.
AI is the “Next Computer” – But with a Twist
AI certainly has the potential to be as transformative as computing once was, but it’s not the same straightforward tool that the computer was. While the computer transformed business and personal productivity by making data accessible and automating basic processes, AI goes further, promising to emulate human reasoning and decision-making. If the computer was a tool for organizing information, AI aspires to be an assistant that can make decisions, respond to real-time scenarios, and adapt to complex environments.
Much like System 360 established standards for interoperability and scalability, the AI ecosystem we’re building today is laying down its own infrastructure, from data pipelines to ethical guidelines and deployment frameworks. But here’s where the twist comes in: unlike System 360, which built on already-established foundations, AI is still a young, fragmented field. Today’s efforts are as much about understanding the technology itself as they are about implementing it. Ethical concerns, data quality issues, and infrastructure needs are all potential hurdles that could slow AI’s progress, especially as society grapples with the implications of highly autonomous systems.
If AI succeeds in becoming a foundational technology, it will redefine entire industries and create new types of jobs, just as computing did in the 1960s. It can be argued that the challenges are much steeper, and the future more uncertain and that, unlike System 360, AI may not find universal success or acceptance, and its journey to societal integration could face more hurdles than IBM ever anticipated. And sure, it's a big bet. But having lived through a number of minor and major technological shifts I think I can say, with some confidence, that anyone who ignores the current trajectory does so at their own peril.
The Next Developer
One of the most exciting parallels to IBM’s story is the potential for a new kind of developer. Just as IBM’s early developers came from varied backgrounds—math, physics, statistics—AI could similarly benefit from a more diverse talent pool. After all, AI doesn’t just need people who can code; it needs people who understand psychology, ethics, domain-specific applications, and even human behavior.
But while the idea of a new kind of developer is appealing, it’s far from simple to put into practice. Training individuals from non-CS backgrounds to work effectively in AI requires an education system capable of supporting that transition, which is no small feat. While the first generation will be recruited and trained internally, universities and companies alike will need to invest in creating interdisciplinary programs that combine technical skills with domain expertise. And not every field will be a natural fit for AI work; the learning curve remains steep, and reskilling non-technical individuals to work with cutting edge tools isn’t a straightforward process. The optimism around a diverse AI workforce needs to be tempered with realism—interdisciplinary teams are valuable but also complex to build and maintain.
In reality, while non-CS fields like psychology, ethics, and domain expertise can contribute to AI, the core technical demands will still require specialized skills. AI development, at least in the foreseeable future, will require a blend of high-level technical competencies in data science, machine learning, and model training. While diverse expertise will enrich the field, we shouldn’t underestimate the technical barriers that will still define the role of “AI developer.”
And while AI will undoubtedly open doors to new types of professionals, but technical skills will still matter. Future developers will likely come from varied backgrounds, yes, but they’ll need to blend domain expertise with a working understanding of AI. We may see an influx of people from humanities, social sciences, and other non-technical fields, but their contribution will require significant training, and they may work alongside, rather than replace, traditional developers.
For instance, a historian or psychologist working in AI may not be developing models directly but instead advising on areas like cultural sensitivity, user behavior, or ethical considerations. In this way, AI could indeed be shaped by a more diverse array of professionals, but the role of traditional technical skills should not be underestimated. While AI may democratize access to technology, true innovation in AI will still depend on a deep understanding of complex models and algorithms.
Challenges Ahead
Perhaps one of the most understated aspects of the AI conversation is the importance of regulation and data quality. AI systems are only as good as the data they’re trained on, and current data sources are often biased, incomplete, or unreliable. Unlike the early days of computing, where improvements in hardware and processing were enough to fuel growth, AI’s progress depends heavily on improving data pipelines and addressing inherent biases. And as AI becomes more prevalent, regulatory bodies are likely to impose restrictions on how it can be used, potentially slowing its adoption in some fields.
Infrastructure, too, is a significant hurdle. AI demands massive computational power, and the cost of maintaining AI models is prohibitive for many organizations. Without a clear path for developing sustainable AI infrastructure, widespread adoption could be limited to organizations with the financial resources to support it. In this way, the AI revolution could face more barriers than the computing revolution, as society grapples with issues like data privacy, ethical usage, and the environmental impact of large-scale AI models.
So The Future is Bright, Maybe
Looking back at IBM’s 1965 gamble, it’s tempting to draw direct parallels to today’s AI investments. While AI certainly has the potential to be a foundational technology, it’s not a simple repeat of 1965. The challenges—ethical, technical, and logistical—are more complex, and the field’s future is less certain. AI could reshape society as computing once did, but it’s equally possible that it will face insurmountable challenges that slow its progress or limit its applications.
For those who dream of AI transforming every industry, there’s plenty to be optimistic about. But we must be prepared to address the realities of data quality, regulatory hurdles, and the complexities of interdisciplinary collaboration. If AI succeeds, it will require more than just technical brilliance; it will need an industry willing to confront its limitations and adapt responsibly.
As for the next generation of developers, they’ll be a fascinating mix of backgrounds, but they’ll also face the challenge of balancing technical demands with ethical considerations and societal expectations. AI’s future is bright, but it’s not straightforward. The real test will be whether we can shape this technology thoughtfully, guided by the lessons of the past and the complexities of the present.